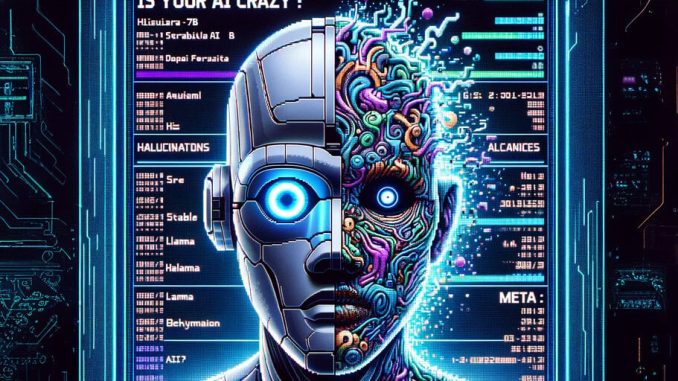
For all the transformative and disruptive power attributed to the rise of artificial intelligence, the Achilles’ heel of generative AI remains its tendency to make things up.
The tendency of Large Language Models (LLMs) to “hallucinate” comes with all sorts of pitfalls, sowing the seeds of misinformation. The sphere of Natural Language Processing (NLP) can be dangerous, especially when people cannot tell the difference between what is human and what is AI generated.
To get a handle on the situation, Huggingface—which claims to be the world’s largest Open Source AI community—introduced the Hallucinations Leaderboard, a new ranking dedicated to evaluating open source LLMs and their tendency to generate hallucinated content by running them through a set of different benchmarks tailored for in-context learning.
“This initiative wants to aid researchers and engineers in identifying the most reliable models, and potentially drive the development of LLMs towards more accurate and faithful language generation,” the leaderboard developers explained.
The spectrum of hallucinations in LLMs breaks down into two distinct categories: factuality and faithfulness. Factual hallucinations are when the content contradicts verifiable real-world facts. An example of such a discrepancy could be a model inaccurately proclaiming that Bitcoin has 100 million tokens instead of just 23 million. Faithful hallucinations, on the other hand, emerge when the generated content deviates from the user’s explicit instructions or the established context, leading to potential inaccuracies in critical domains such as news summarization or historical analysis. On this front, the model generates fake information because it seems like it’s the most logical path according to its prompt.
The leaderboard uses EleutherAI’s Language Model Evaluation Harness to conduct a thorough zero-shot and few-shot language model evaluation across a variety of tasks. These tasks are designed to test how good a model behaves. In general terms, every test gives a score based on the LLM’s performance, then these results are averaged so each model competes based on its overall performance across all tests.
So which LLM architecture is the least crazy of the bunch?
Based on the preliminary results from the Hallucinations Leaderboard, the models that exhibit fewer hallucinations—and hence rank among the best—include Meow (Based on Solar), Stability AI’s Stable Beluga, and Meta’s LlaMA-2. However some models that part from a common base (like those based in Mistral LLMs) tend to outperform its competitors on specific tests —which must be taken into consideration based on the nature of the tast that each user may have in mind.
On the Hallucinations Leaderboard, a higher average score for a model indicates a lower propensity for the model to hallucinate. This means the model is more accurate and reliable in generating content that aligns with factual information and adheres to the user’s input or the given context.
However, it’s important to note that models that are great at some tasks may be underwhelming at others, so the ranking is based on an average between all the benchmarks, which tested different areas like summarization, fact-checking, reading comprehension and self consistency among others.
Dr. Pasquale Minervini, the architect behind the Hallucination’s Leaderboard, did not immediately respond to a request for comment from Decrypt.
It’s worth noting that while the Hallucinations Leaderboard offers a comprehensive evaluation of open-source models, closed-source models have not yet undergone this rigorous testing. Given the testing protocol and the proprietary restrictions of commercial models, however, Hallucinations Leaderboard scoring seems unlikely.
Edited by Ryan Ozawa.
Be the first to comment